Data Analysis and Interpretation Presentation
Data Analysis and Interpretation Presentation
using the article uploaded, please prepare 4 slides about the advantages and drawbacks associated with the statistical significance research method. Write speaker notes to elaborate.
Statistical significance tests: Equivalence and reverse tests should reduce misinterpretation
Author: Parkhurst, David F
Publication info: Bioscience 51. 12 (Dec 2001): 1051-1057.
Abstract: Because both biological phenomena and the measurements researchers make in studying them are often quite variable, methods of statistical analysis have much to offer science. Equivalence tests improve the logic of significance testing when demonstrating similarity is important, and reverse tests can help show that failure to reject a null hypothesis does not support that hypothesis.
Headnote
EQUIVALENCE TESTS IMPROVE THE LOGIC OF SIGNIFICANCE TESTING WHEN DEMONSTRATING SIMILARITY IS IMPORTANT, AND REVERSE TESTS CAN HELP SHOW THAT FAILURE TO REJECT A NULL HYPOTHESIS DOES NOT SUPPORT THAT HYPOTHESIS
Because both biological phenomena and the measurements researchers make in studying them are often quite variable, methods of statistical analysis have much to offer science. Statistical analysis has many aspects, including describing distributions of data, exploring the fit of data to various models, estimating and determining confidence intervals for parameters such as means and variances, and helping to identify real effects in the face of random variation (separating signal from noise). Significance tests, a tool for the latter purpose, are the focus of this article. Sometimes it seems that many biologists view significance testing as the overriding purpose of statistics, although many statisticians (Chatfield 1985) lament the overemphasis on that aspect of their trade at the expense of other aspects.
To view significance tests at their most useful, consider the following scenario: An inebriated guest at a party claims the ability to influence the outcome of coin flips. Upon your challenge for evidence, he suggests that you and he go to separate rooms, with each of you accompanied by a witness of your choosing. You are to wait 5 minutes while he writes down a sequence of 10 “H” and “T” letters and then flip a coin (supplied by you) 10 times and record the outcomes. During the wait, you decide you will analyze the results with a significance test, with the null hypothesis being that he is fundamentally no better than a 50:50 guesser, and the one-tailed alternative being that he really has at least some ability to match more than 50% of coin tosses in this way.
Suppose the sequence he writes is HTTHTTTTHT, and your flips turn out as HTHHTTTTHT, so that 9 of the 10 match. From the binomial distribution, the probability of his matching 9 or 10 tosses correctly out of 10, if he were really only a 50% guesser, is just under 0.011. If you had decided to use the conventional alpha = 0.05 (i.e., a 5% probability of rejecting the null hypothesis if it were really true), then you would have to reject the null hypothesis and consider the result to be evidence for the fellow’s claim. However, if you had been really skeptical of the claim, and if having to believe him would be costly (to your peace of mind, perhaps), then you might have chosen to require a smaller alpha (0.01 or even smaller); then you would not have had to reject the null hypothesis.
The point of this example is that significance tests make some sense in situations for which there is good reason, if only Ockham’s razor (Jeffreys and Berger 1992), to believe a null hypothesis, and we wish to place a strong burden of proof on those who would attempt to refute that hypothesis. Many scientific investigations are not of this form, however, so significance testing is often not the most appropriate way to analyze data. For example, it often makes more sense to estimate how large some effect is, rather than simply to determine whether the effect occurs (Tukey 1991).
When significance tests are used and a null hypothesis is not rejected, a major problem often arises -namely, the result may be interpreted, without a logical basis, as providing evidence for the null hypothesis. This illogical misinterpretation can be a problem for two distinct reasons:
1. Especially in many applied situations, the most relevant question may be whether the responses of some result Y to treatments X1 and X2 are similar enough for practical purposes, rather than whether the responses to the two treatments are different. Yet significance tests of the common form with no-effect null hypotheses address the second type of question, not the first, and failure to reject a no-effect null hypothesis does not provide evidence that the two treatments are “similar enough” in their effects on Y.
2. As illustrated by the coin example, traditional significance testing has the effect of placing a burden of proof on those who wish to claim that a factor X does influence a response Y. If we assume that the goal of basic science is to work toward determining what is true, then it seems we should require equally strong proof from those who would state that factor X does not affect response Y. However, biologists and other scientists commonly report results that are not “statistically significant,” using statements like “X did not affect Y” without providing evidence for the lack of an effect. Audiences or readers then seem prone to accept such statements without asking for evidence that X really did not affect Y.
A large and growing literature describes numerous other problems with significance testing. Hunter and Schmidt (1997) and Anderson and colleagues (2000) provide good leads into that literature. Useful replacements exist, including emphasis on estimates and associated indicators of precision such as confidence limits (Gardner and Altman 1986, Poole 2001), Bayesian analysis (Box and Tiao 1973, Jeffreys and Berger 1992, Ellison 1996), likelihood analysis (Royall 1997), and methods based on information theory (Anderson et al. 2000). This article focuses on the two particular problems just described.
Applied science. Two examples of “accepting the null hypothesis”
Example A1. (This example is based loosely on a real occurrence from 1999.) Researchers interested in controlling insect species V, a vector for a disease that infects various mammals, including humans, perform a pilot study to test whether spraying a certain fungus in forests will reduce the numbers of the vector species. Potential changes in populations of nontarget species that might be caused by the fungus are also of concern, and this example focuses on those effects.
The researchers lay out 30 blocks at random locations in some forested land and spray the fungus on half of each block. One week later, 20 soil cores are collected from each half block, and the numbers of mites (one of the nontarget taxa of interest) extracted from the combined (composited) soil from each half block are counted. With the use of conventional significance testing, the researchers then perform a paired t test for the no-effect null hypothesis H^sub o^ (no difference in population mean numbers of mites between the sprayed and unsprayed areas) versus H^sub alpha^ (fewer or more mites on average in sprayed areas). Suppose that the data appeared to be roughly log-normal in distribution and were log transformed to provide a test of the ratio of mite numbers in the treated area to numbers in the control area.
Suppose next that about 7% fewer mites were collected on average from the sprayed subplots, but, because of the variability in the paired differences, this result was “not statistically significant” at alpha = 0.05 (P = 0.22, say). It might then be argued by some scientists or decisionmakers that “the fungus had no effect on the mites,” and that result might mistakenly be taken as an indication of the safety of the fungus to those organisms. However, failure to reject a null hypothesis of no difference does not justify such an interpretation, for as Sagan (1995, p. 213) has noted, “Absence of evidence is not evidence of absence [of some phenomenon].”
If protection of mites and other nontarget taxa is considered important in a situation like this (as I believe it should be), then the question that should be emphasized is “Do we have evidence that the fungus is safe for the mites?” rather than the much weaker “Do we lack evidence that the fungus is not safe for the mites?” This distinction is important because of the ways that significance tests, with the sample sizes and degrees of variability that often apply in biological studies, make rejection of null hypotheses generally difficult and failure to reject null hypotheses relatively easy. The equivalence tests to be described below allow asking directly for evidence of safety, and they remove the need to treat lack of evidence for undesirable effects illogically as evidence for safety. As McBride (1999) has suggested, reversing the burden of proof in this way is consistent with the “precautionary principle.”
Example A2. More generally, “safe” levels for toxic chemicals are often set by determining so-called lowest-observedeffect levels (LOELs) and no-observed-effect levels (NOELs). However, LOELs are not determined by direct observation, but rather as “the lowest toxicant [level] in which the values of the measured response are statistically significantly different from those in the control” (Greenberg et al. 1992, p. 8-2). The NOEL is then the next lowest toxicant level, at which no statistically significant response had been observed. This does not mean that the toxicant had no important biological effect at that level, but only that a different response from the control had not been “proven beyond reasonable doubt,” so to speak. Stephan and Rogers (1985) and Suter (1996), among others, have argued against this biased way of choosing levels to ensure safety, but the method is still commonly used.
The equivalence tests described later can help to improve the contribution of statistics in applied situations like these by allowing questions about similarity (and relative safety) to be addressed directly rather than in a backhanded way.
Basic science. Three examples of “acce tint the null hypothesis”
Example B1. I have twice reviewed papers in which authors argued in essence that because the mean of some group X^sup 1^ was not “significantly” different from the mean of a group X^sup 2^, the factor X could have no effect on some response Y (Parkhurst 1985). As a result, in both cases the authors proposed to neglect factor X and to study only the effects of other factors on Y. It is clear that significance testing causes some scientists to misunderstand their data and to learn wrong things from those data. Surely this misunderstanding slows scientific progress (Schmidt 1996). This attitude may also lead authors not to submit, or reviewers to reject, papers on results that are not “statistically significant” (Csada et al. 1996, Bauchau 1997).
Example B2. As noted previously, failure to find statistical significance for “X affects Y” is often reported in talks and papers with an assertion that X does not affect Y. For example, Hobbs (1988) reported that “structurally heterogeneous patches are not more species rich than their homogeneous counterparts” (p. 149; emphasis added). However, the data provided there suggested otherwise. The mean species richness in 10 heterogeneous patches varied from 13% to 37% higher than that in the 20 homogeneous patches studied, depending on whether the data were combined overall, divided into native versus alien classes, or divided by growth form (trees, shrubs, or herbs). The ratio was 1.20 for all species combined. The P values for the six comparisons ranged from about 0.19 to 0.39. Thus, while it may be true that the data do not provide “evidence beyond reasonable doubt” (at alpha = 5% or even 10%) that species richness differed between the two treatments, there is certainly no convincing evidence that it did not so differ. In the interests of truth in science, authors should avoid claiming lack of effects unless they perform equivalence or reverse tests like those to be described later.
Example B3. I have read many papers with similar claims of “no effect,” and I have often heard such claims in talks, including talks at every meeting of the Ecological Society of America that I have attended in the past 20 years. At times I have asked speakers, in private conversations after their talks, why they had asserted “no effect” when a hypothesized effect (or occasionally its opposite) seemed to be indicated in a table or plot of the data. I have received two types of answers: Some have replied, “Well, you know what I mean. What I said was just shorthand for `no statistically significant effect:” Others have answered thus: “Well, it wasn’t statistically significant, so whatever effect I saw must have resulted only from random variation,” implying that they had concluded that their null hypotheses were true at the population level.
With the first of these replies, not every listener or reader of a paper, including journalists who may report on the researcher’s work and decisionmakers who may make use of the reported work, will understand the difference between what was said and what was meant. I suggest that “truth in science” requires us not to use code words with hidden, jargonistic meanings.
The second way of thinking is the (il)logical equivalent of failing to find a pair of pliers in a quick search of a messy garage and claiming that failure to be good evidence that the pliers were not there. This interpretation may be even more problematic than the first, suggesting as it does that the scientist believed that lack of a statistically significant result should be treated as positive evidence for lack of an effect at the population level. In more than 20 years of teaching graduate statistics, I have had numerous students who have said they had been taught to interpret lack of statistical significance in that way. Clearly, if some listeners or readers take “no effect” statements literally, then others who use such statements as shorthand will at times be misunderstood. Hunter and Schmidt (1997) indicate that many psychologists share this kind of misinterpretation.
Between these two types of response lies a third form of confusion, that is, the notion that if an effect is not “significant” then it is not important. As a related point, over the years I have reviewed numerous ecological papers in which both very different meanings of the word “significant”-statistically detectable versus substantially important-were used in the same paper. Very often when that is the case, there is no indication that the authors had been dear in their own minds which meaning they were implying at any given time, and, even if they had been, the two different meanings would most likely confuse readers.
Some textbooks do a good job of describing the correct logic associated with nonrejection, but others do not. For example, Samuels (1989, p. 209) wrote, “In other words, nonrejection of H^sub o^ is not the same as acceptance of H^sup o^…. Nonrejection of H^sub o^ indicates that the data are compatible with H^sub o^ , but the data may also be quite compatible with H^sub A^ .” The reverse tests described in the next section serve to demonstrate to both the producers and the users of data that failing to demonstrate some effect statistically is not equivalent to demonstrating that the effect does not occur.
Equivalence and reverse tests, and how they can help
In this section, I describe a method for looking at data statistically but with different null and alternative hypotheses from the no-effect null hypotheses that have been used most often to date. In many situations in which data are obtained to help decisionmakers choose among various actions, it will be useful to have tests to determine whether a difference between two or more treatments is small enough to allow the treatments to be considered equivalent in a practical sense. Such tests, which pharmacologists have used extensively to help determine whether two drug formulations have similar enough physiological and therapeutic effects, are often termed bioequivalence or, more generally, equivalence tests.
These tests can also be used (especially in basic science) in a second way, for which I use the term reverse tests. In this form of use, they are applied after a no-effect hypothesis test has failed to yield a “significant” result. Then, the reverse test can help in determining whether the original result could reasonably be interpreted as demonstrating lack of an important response, or whether it should more accurately be taken as evidence for lack of certainty caused by some combination of high variability and inadequate sample size.
Mechanics of equivalence and reverse tests This section provides examples of equivalence tests and reverse tests. Equivalence tests are appropriate when demonstrating similarity rather than differences in responses is desirable, and reverse tests can help in deciding whether data that fail to provide statistical evidence for a difference can be used to infer lack of any important practical difference.
Relationship to power analysis
Some readers may notice a relationship between the ideas described here and the concept of power of a statistical test-that is, the probability that the null hypothesis would be rejected if that hypothesis were wrong by a certain amount. Estimates of power are especially useful in the research planning stage, when they can be used to predict what sample sizes will be needed to provide a given degree of power in the analyses planned for the data one expects to obtain. However, consider a situation in which a no-effect null hypothesis has not been rejected. In such a situation, a reverse test to check whether the data provide evidence that “whatever effect is there is smaller than some minimum important effect size” seems fairly easy to interpret. In my experience, it has seemed less useful in such circumstances to estimate how often the null hypothesis would be rejected if the true effect size were equal to that MIES and if the experiment could be repeated many times. Post hoc power analysis has also been questioned by others (e.g., Hoenig and Heisey 2001 and references therein).
Conclusions
In my own experience, in spite of my teaching about interpretation of significance tests in statistics classes, on final examinations students frequently expressed the belief that failing to reject a null hypothesis meant that the hypothesis was true. Since I have begun teaching reverse tests, the proportion of students making this error has declined dramatically.
In December 1996, a task force for the American Psychological Association (APA) met to consider, among other issues, whether statistical significance tests should be banned from APA journals (Azar 1997). The group did not ultimately recommend such a ban, but formation of that task force indicates the existence of serious problems with some of the ways significance testing is commonly used in science. This article has concentrated on two related subsets of those problems and suggested some methods, unfamiliar to many biologists, for reducing the occurrence of those problems. Specifically, when the logic of a situation calls for demonstration of similarity rather than differences among responses to various treatments, then equivalence tests are often more relevant than tests with traditional no-effect null hypotheses (Anderson and Hauck 1986, Dixon 1998). In other situations for which the traditional test is thought to be useful, but in which the null hypothesis is not rejected, reverse tests can be used to help sort out whether any effect that may exist is really close to negligible or whether (as is often the case) the results must simply be treated as inconclusive.
The crux of the problem appears to be that users of hypothesis tests often seem to believe, and perhaps to wish, that the tests can distinguish between “the null hypothesis is true” and “the alternative hypothesis is true.’ The methods outlined here help to make dear that tests can at most distinguish between “the null hypothesis seems likely to be false” and “given our data, we cannot tell which hypothesis is correct.’ For statistical methods to aid in scientific progress, they must be interpreted correctly.
We biologists have conflicting goals when interpreting data: We should avoid the all-too-human tendency to see what we are looking for and to miss seeing what we are not expecting. At the same time, we should not be misled into thinking that a statistical hypothesis test can show that either the null hypothesis is false and the alternative true, or vice versa. In fact, significance tests can at best provide evidence against the null hypothesis, but never for it (unless it is known that power is sufficiently high). Significance testing is popular because it can help prevent treating data that may represent random variation around occurrences expected if the null hypothesis is true as evidence for the alternative. It is time to adopt further procedures, like equivalence and reverse tests, to prevent the equally undesirable action of treating a no-effect null hypothesis as true, simply because it could not be rejected. We need to be more willing to acknowledge that a study has been inconclusive.
Acknowledgments
I thank Margaret Carreiro, Philip Dixon, the late Bernard Flury, Charles Fox, Ellen Ketterson, Richard Pouyat, and John Wehr for helpful discussions and advice on this and an earlier version of this article.
AuthorAffiliation
David F. Parkhurst (email: [email protected]), a physiological plant ecologist, is professor of environmental science in the Environmental Science Research Center, School of Public and Environmental Affairs, Indiana University, Bloomington, IN 47405. His research interests are in environmental statistics and effective use of statistics in science.
Subject: Biology; Statistical analysis; Logic; Hypotheses; Research
Publication title: Bioscience
Volume: 51
Issue: 12
Pages: 1051-1057
Number of pages: 7
Publication year: 2001
Publication date: Dec 2001
Year: 2001
Publisher: University of California Press
Place of publication: Washington
Country of publication: United States
Journal subject: Agriculture, Biology, Medical Sciences
ISSN: 00063568
CODEN: BISNAS
Source type: Scholarly Journals
Language of publication: English
Document type: Feature
ProQuest document ID: 216467333
Document URL: http://search.proquest.com/docview/216467333?accountid=35812
Copyright: Copyright American Institute of Biological Sciences Dec 2001
Last updated: 2011-09-12
Database: ProQuest Biology Journals; ProQuest Central; ProQuest Education Journals; ProQuest Research Library;
ORDER THIS ESSAY HERE NOW AND GET A DISCOUNT !!!
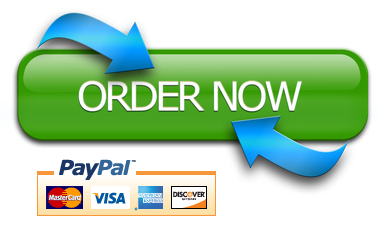
You can place an order similar to this with us. You are assured of an authentic custom paper delivered within the given deadline besides our 24/7 customer support all through.
Latest completed orders:
# | topic title | discipline | academic level | pages | delivered |
---|---|---|---|---|---|
6
|
Writer's choice
|
Business
|
University
|
2
|
1 hour 32 min
|
7
|
Wise Approach to
|
Philosophy
|
College
|
2
|
2 hours 19 min
|
8
|
1980's and 1990
|
History
|
College
|
3
|
2 hours 20 min
|
9
|
pick the best topic
|
Finance
|
School
|
2
|
2 hours 27 min
|
10
|
finance for leisure
|
Finance
|
University
|
12
|
2 hours 36 min
|